
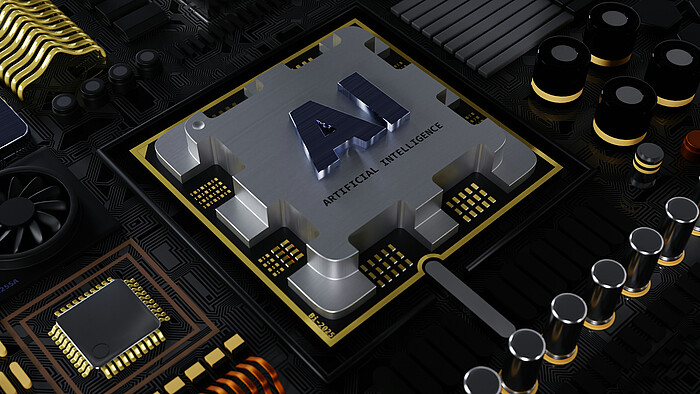
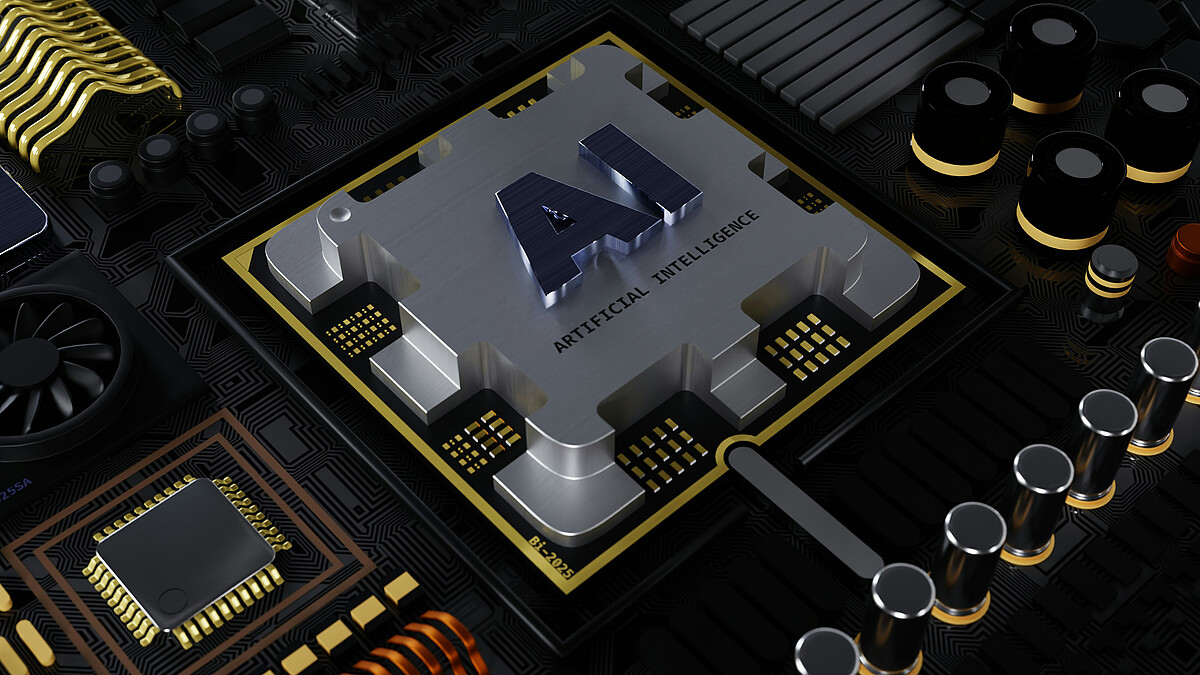
20th November 2024
Workshop on Insurance and Financial Mathematics:
Machine Learning, Artificial Intelligence, and Insurance
20th November 2024
Workshop on Insurance and Financial Mathematics:
Machine Learning, Artificial Intelligence, and Insurance
Abstracts
-
Markus Gesmann: Hierarchical Compartmental Reserving Models
Hierarchical compartmental reserving models provide a parametric framework for describing the high-level business processes driving claims development in insurance using differential equations.
We will discuss how those models can be presented in a fully Bayesian modelling framework for the aggregated claims settlement process to capture trends observed in paid and outstanding claims development data reflecting the random nature of claims and latent underlying process parameters.
We show how the experienced modeller can utilise her expertise to describe the volatility of the underlying risk exposure profile and uncertainty on prior parameter assumptions and highlight in particular the subtle, but important difference between modelling incremental and cumulative claims payments. -
Marius Lindauer: The Transformative Impact of Modern Generative AI and the Role of AutoML in AI's Advancement
The emergence of modern generative artificial intelligence (genAI) has revolutionized the field of AI, offering unprecedented capabilities in generating human-like text, images, and other complex data forms. This talk delves into the importance of genAI in today's technological landscape, highlighting its transformative effects on industries such as healthcare, finance, and creative arts. We provide a technical overview of key concepts that underpin these advancements. Additionally, we explore the potential of Automated Machine Learning (AutoML) within this context. AutoML promises to democratize AI by automating the design and optimization of models, thus lowering the barrier to entry and accelerating innovation. By integrating AutoML with AI, we can enhance model performance, reduce development time, and foster broader adoption across diverse sectors.
-
Peyman Mohajerin Esfahani: The Role of Convexity in Data-Driven Decision-Making
In this talk, we study a general class of data-driven decision-making problems and discuss different terminologies and research questions that emerge in this context. As a decision mechanism (the mapping from data to decisions), we introduce a broad class of data-driven optimization known as distributionally robust optimization. We then highlight three different aspects of computation, statistics, and real-time implementation of these problems, and elaborate on how convexity can help in each of these aspects. A particular focus will be given to real-time implementation, which is closely connected to the topic of Online Optimization.
-
Matthias Fahrenwaldt : Supervisory perspective on the use of generative AI
Generative AI is increasingly used in the financial sector. The main applications at insurance companies include claims management and, more generally, increasing efficiency in operations. The use of generative AI also entails risks that can be clustered in terms of governance, IT/outsourcing, mathematical methodology, and the use of AI in decision processes. This talk addresses these risks and discusses them from a regulatory perspective.